Structure From Action
Learning Interactions for Articulated Object 3D Structure Discovery
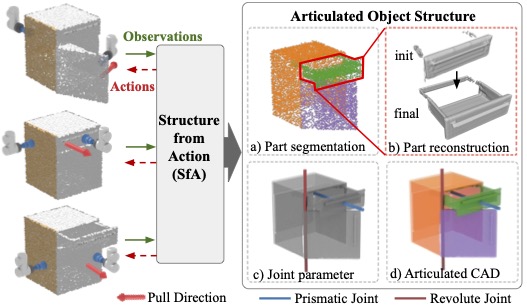
We introduce Structure from Action (SfA), a framework to discover 3D part geometry and joint parameters of unseen articulated objects via a sequence of inferred interactions. Our key insight is that 3D interaction and perception should be considered in conjunction to construct 3D articulated CAD models, especially for categories not seen during training. By selecting informative interactions, SfA discovers parts and reveals occluded surfaces, like the inside of a closed drawer. By aggregating visual observations in 3D, SfA accurately segments multiple parts, reconstructs part geometry, and infers all joint parameters in a canonical coordinate frame. Our experiments demonstrate that a SfA model trained in simulation can generalize to many unseen object categories with unknown kinematic structures and to real-world objects. Empirically, SfA outperforms a pipeline of state-of-the-art components by 25.0 3D IoU points. Code and data will be publicly available.
Real World Experiments
To demonstrate the feasibility of SfA in the real-world setting, we set up a single-arm tabletop environment. The robot arm is equipped with a cylindrical pusher, which moves the object parts based on the inferred actions. The environment has 4 Intel RealSense RGBD cameras, together capturing a RGB point cloud of the object. The interaction and 3D reconstruction results are shown in the video below. We believe the real-world results show that SfA is a promising step in future interactive perception and robotics research.
Method Summary
As an overview, we contrast our method with recent work. Our goal is to recover objects' articulation structure, including objects' part reconstruction , segmentation , and joints estimation. An algorithm should also handle objects with multiple (Multi.) parts. While prior work tackles some of these challenges, SfA presents a comprehensive framework addressing all facets of the problem.
Given a raw RGB point cloud, SfA infers and executes informative actions to construct an articulated CAD model, which consists of multiple 3D part meshes and the revolute, and prismatic joints connecting them. The SfA framework consists of four components: an interaction policy, which chooses informative actions that move parts, a part aggregation module, which tracks part discoveries over a sequence of interactions, a joint estimation module, which predicts joint parameters and kinematic constraints of the articulation, and finally, the pipeline for the construction of the articulated CAD model.
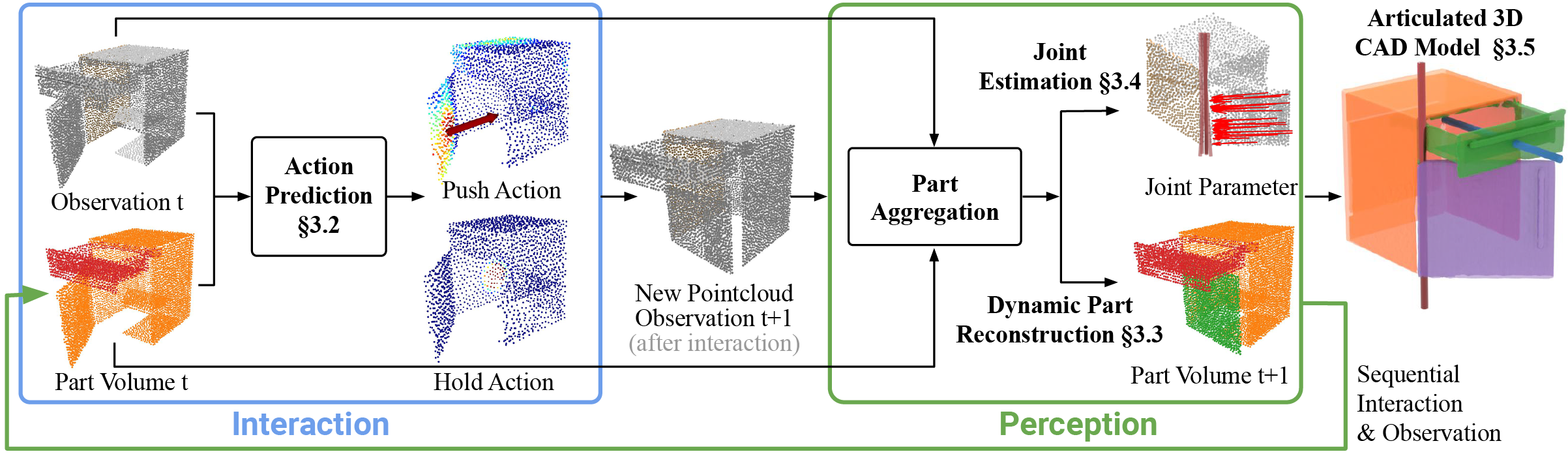
SfA Interaction and Perception Pipeline
The video below demonstrates SfA's ability to infer informative multi-step interactions given an articulated object, and generate the articulated 3D CAD model of the object overtime. By first inferring the push and hold actions, and then inferring the parts reconstruction and joint parameters, SfA is able to construct the full 3D articulated CAD model in 3 steps.
3D Articulated CAD Models Results
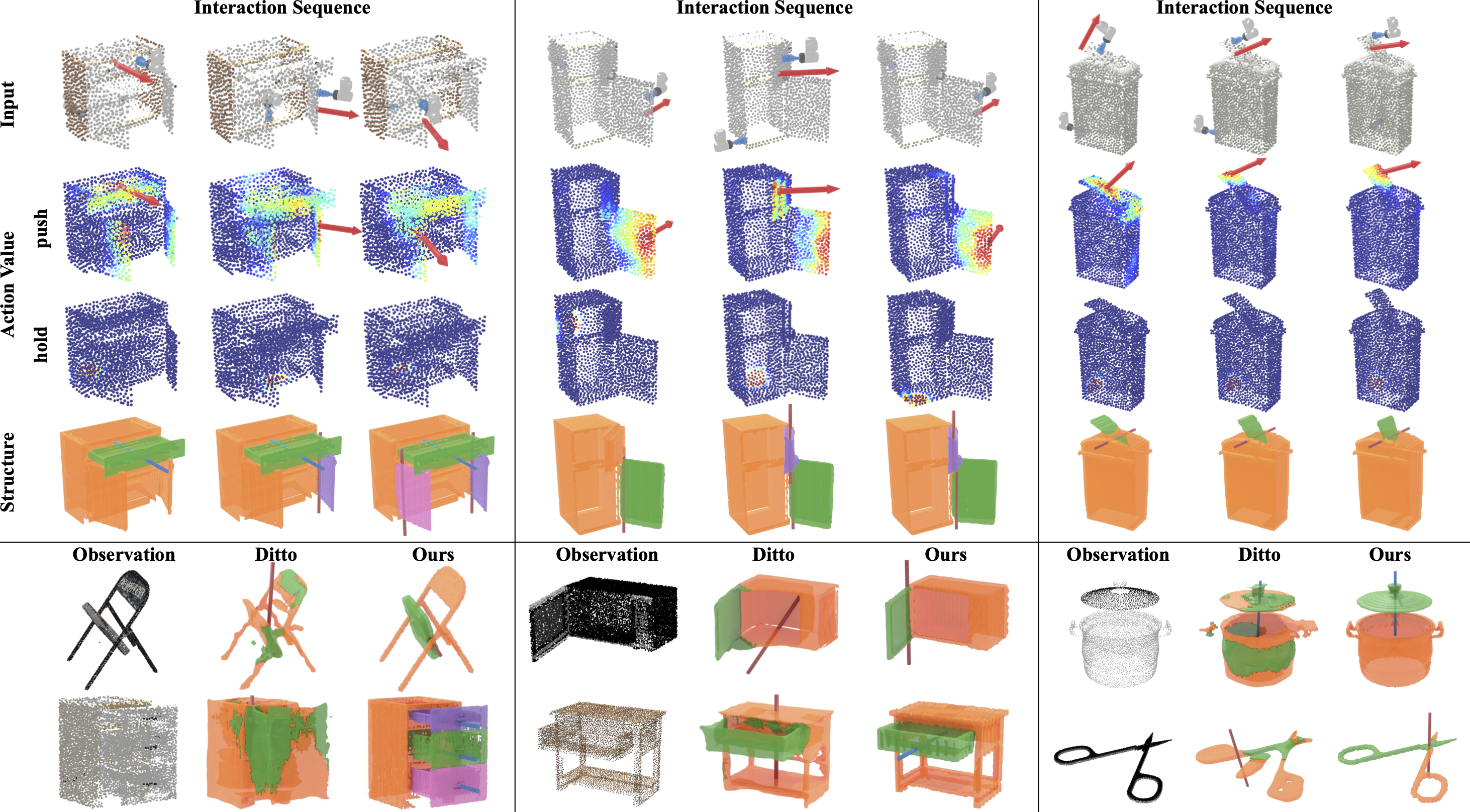
On the top, we show the step-by-step results from the SfA pipeline. The inferred actions prioritize new parts discovery and expose articulations. Below we show SfA 3D reconstruction result on unseen objects with different shapes, sizes, and kinematic structures. The pipeline can handle both large (furniture) and small (scissor) objects, as well as prismatic (drawers, pots) and revolute (microwave, chair) joints. Our method outperforms the Ditto on both parts reconstruction and joints estimation (revolute: red, prismatic: blue).
We show the complete and animated 3D articulated CAD model from the SfA pipeline.
Paper
Latest version: arXiv: [cs.CV] or here
Code and instructions will be avaliable.
Team
Acknowledgements
This work was supported in part by National Science Foundation under 2143601, 2037101, and 2132519. Thank you Cheng Chi, Huy Ha, Zhenjia Xu, Zeyi Liu, and other colleagues of the CAIR lab for your valuable feedback and support. Thanks to Cheng Chi and Zhenjia Xu for your help with the UR5 robot experiments. We would like to thank Google for the UR5 robot hardware.
Contact
If you have any questions, please feel free to contact Neil
Bibtex
@article{nie2022sfa,
title={Structure from Action: Learning Interactions for Articulated Object 3D Structure Discovery},
author={Nie, Neil and Gadre, Samir Yitzhak and Ehsani, Kiana and Song, Shuran},
journal={arxiv},
year={2022} }